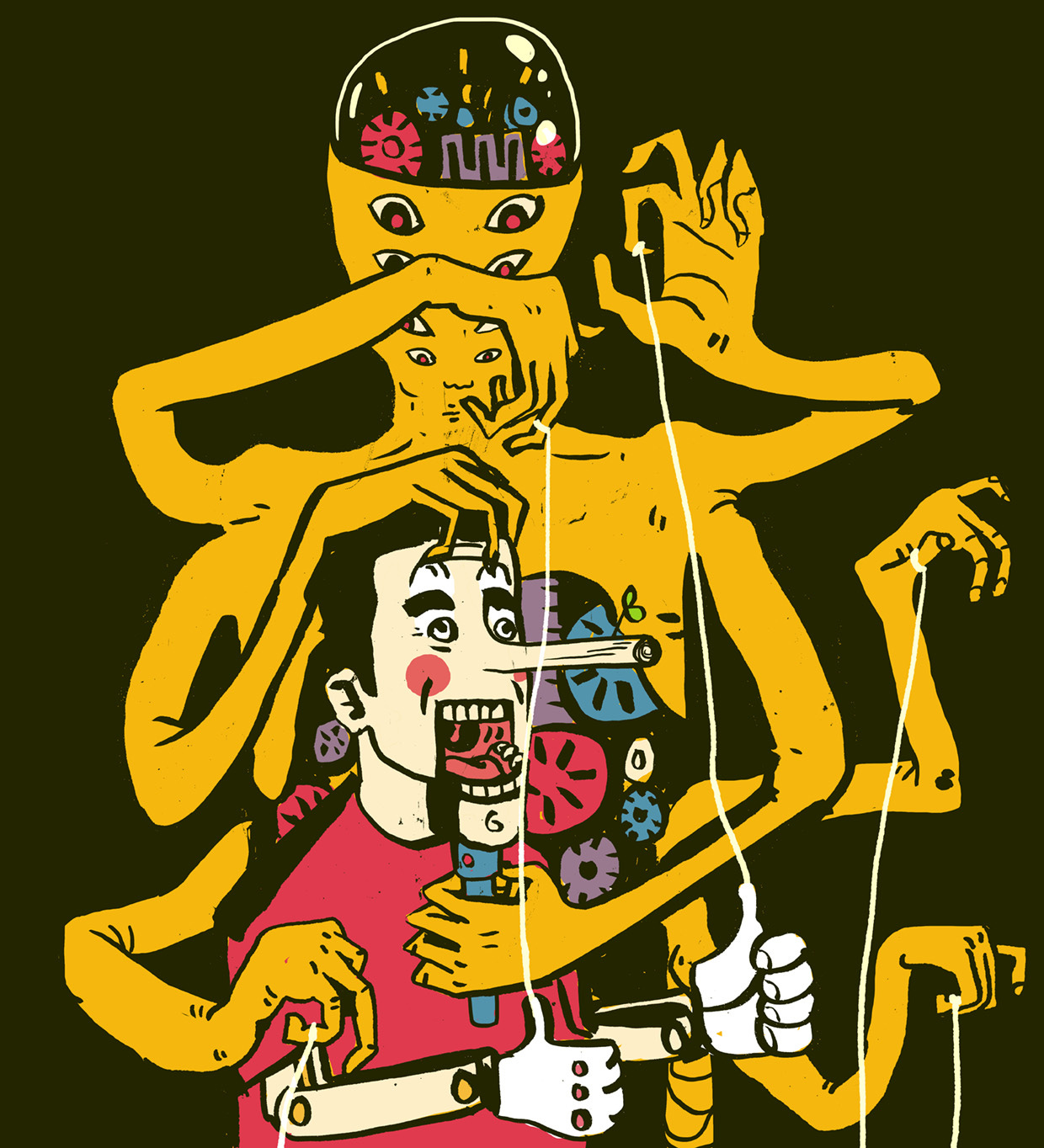
The future is already here, and becoming more evenly distributed every day. Just back in 2006, when I first went off to college, the field of AI was, academically, considered a joke. And some people, somehow, still see it this way, even as almost every day new AIs are released that cost millions to train and demonstrate ever more impressive abilities. The key advancement is that new AIs can do a multitude of different tasks well, instead of being hyper-specialized. In this they are a form of early proto-AGI: artificial general intelligence, considered the holy grail of the field. These AIs have the ability to write convincing essays about many subjects, or play hundreds of types of games, or create AI-artwork of almost anything imaginable at the click of a button, and come bearing techno-angelic names like PaLM, Gato, GPT-3, DALL-E 2, Imagen, etc.
In response to these new AI models there is a rising class of AI critics, who downplay the results by focusing on terminology, fuzzy benchmarks, and minimization—and if the tide takes down your sandcastle, just build it further up the beach.
Most disagreements in a highly abstract field break down into quibbling over language. Wittgenstein showed us that. His proposal was that thinkers become doctors of language, that they track down the swelling of syntactic lymph nodes, that they administer the orthopedic brace of diagrammatic definitions. And nowhere is a language doctor more necessary than in the contemporary debates around AI.
Consider one of the most vocal critics of AI: Gary Marcus, academic psychologist and once New Yorker writer, now Substacker. He’s hit on this topic after searching around for a few different ones over the years, ranging from a nonfiction book on how he learned to play the guitar to his book on evolutionary psychology, Kluge, from 2008, in which he argues that human brains are terrible cognitive messes and implies on multiple occasions that computers and robots have particular advantages, like having a perfect memory, or having the ability to be more Spock-like and logical. However, Marcus now emphasizes the power of the human mind, and how dumb AIs are in comparison, a view he has repeatedly outlined in recent years in places like The New Yorker, The New York Times, Nautilus, and so on. And I should note: I’ve met Marcus before, twice, albeit briefly each time. I also went to the same undergraduate college and was mentored by the same professors at the exact same School of Cognitive Science. So I know where he’s coming from. And I’m sympathetic to some of his criticisms. For it’s obvious these current AIs don’t have human-like understanding, and sure, it’s not AGI that’s ready to be deployed at scale or reliably. But the role of AI skeptics like Marcus has evolved into perpetually downplaying what are absolutely out-of-this-world achievements, compared to just a decade ago.
Here’s Marcus’ reaction to the new Gato, which DeepMind describes as a
network with the same weights can play Atari, caption images, chat, stack blocks with a real robot arm and much more, deciding based on its context whether to output text, joint torques, button presses, or other tokens.
Marcus dismisses it in its entirety:
This is far from being “intelligent”. . . Nature is trying to tell us something here, which is this doesn’t really work, but the field is so believing its own press clippings that it just can’t see that.
Or consider what he called LaMDA, the chatbot model so advanced it convinced a Google engineer that it was sentient, which Marcus described as a mere “spread-sheet for words.”
In order to justify such round dismissals of something that was flatly impossible a few years ago, Marcus, as do essentially all of AI’s critics, simply shifts the definition of “intelligent” to mean that AIs must not only match humans in terms of ability (i.e, if both were given the same GRE questions), but must also be human-like in how they reason.
I single out Marcus as representative of AI critics everywhere only because he has, very explicitly, embraced being a figurehead for the doubter side of the debate, and is the one who has made the most vocal and standard-bearing arguments; e.g., calling current approaches to AI “fantasy thinking.” Often, science journalists reach out to him for comment about some new AI model, like this recent quote in New Scientist:
These are like parlour tricks. They’re cute, they’re magician’s tricks. They’re able to fool unsophisticated humans who aren’t trained to understand these things.
Or he makes analogies wherein progress in AI is likened to the charade of a horse that knows arithmetic (a comparison he’s made multiple times):
People may no longer believe that horses can do math, but they do want to believe that a new kind of “artificial general intelligence [AGI]”, capable of doing math, understanding human language, and so much more, is here or nearly here. Elon Musk, for example, recently said that it was more likely than not that we would see AGI by 2029 . (I think he is so far I offered to bet him a $100,000 he was wrong; enough of my colleagues agreed with me that within hours they quintupled my bet, to $500,000. Musk didn’t have the guts to accept, which tells you a lot.)
Whence came the money? Marcus’ company was acquired by Uber years ago, and he used his Uber-acquisition wealth to make this $100,000 dollar bet (Marcus then wrote a news story covering his own bet in Fortune magazine). To judge the bet he proposed standards like “AI will not be able to work as a competent cook in an arbitrary kitchen.” Marcus writes to Elon:
Yet, what is such a bet when the goalposts are being ever moved and the terminology always under question?
Let us take what I view as the issue where Marcus is at his best and his criticisms the fairest: self-driving cars. But while he’s right that self-driving cars are not reliable enough for national deployment, due entirely to issues around edge cases, Marcus even objects to the most basic of terminology:
names like AutoSteer, AutoPilot, and Full Self Driving. . . these are still immature technologies that don’t merit such names.
But is it really true that a car that can reliably navigate from arbitrary point A to arbitrary point B through busy city streets doesn’t even deserve the term “AutoPilot?” Or is this an exaggeration of language? A few months ago San Francisco gave out the final permit for driverless robotaxis for the company Cruise, robotaxis that are already deployed and that people ride around the city all the time in. And the same is true in Arizona, wherein Waynmo has been offering rides to the public in driverless taxis for almost 18 months. When I grew up, cars could not drive themselves. Now, cars can definitely drive themselves. I’ve ridden in them, and it’s nigh miraculous.
But the issues of reliability and edge cases matter a lot less for deployment of proto-AGIs, like DALL-E 2, which Marcus criticizes as not being ready to be deployed in a “safety-critical context.” But what matters about DALL-E 2 is how it can produce insanely good and surprisingly knowledgeable images, like being able to illustrate Kermit The Frog in the style of everything from Spirited Away to Blade Runner.
And DALL-E 2 and GPT-3 often evince understanding they shouldn’t. Consider, e.g., how DALL-E 2 can generate sensible images just from emojis.
GPT-3 goes beyond DALL-E 2: it can write faux-Hemingway short stories and sports articles and bland SEO-clickbait, but it can also play Chess and code in multiple programming languages. Back in 2016 nothing like this existed—computers hadn’t even beaten humans at Go! Yet, according to Marcus:
AI is closer to Microsoft Excel than it is to humans. It’s just doing statistical calculations and spitting out a result. It doesn’t actually understand those results.
But GPT-3 is, just objectively, a lot closer to an artificial cortex than it is to Microsoft Excel. Playing around with GPT-3 my jaw dropped on the floor. For Electric Literature I prompted GPT-3 with parts of my novel from last year, The Revelations, along with its book jacket, to see how it would do, and it did a better job than anything trained to merely auto-complete sentences has a right to. This was recently done more experimentally by philosopher Eric Schwitzgebel, who showed that experts could only barely distinguish between the famous philosopher Daniel Dennett’s real answers to questions and GPT-3’s answers.
Nonetheless, it's striking that our fine-tuned GPT-3 could produce outputs sufficiently Dennettlike that experts on Dennett's work had difficulty distinguishing them from Dennett's real answers, and that this could be done mechanically with no meaningful editing or cherry-picking.
While no one thinks GPT-3 could consistently pass a careful and long Turing test by an expert, it’s hard to see how being effectively indistinguishable from one of the most prominent living philosophers is possible if GPT-3 is at all analogous to a horse that does arithmetic. GPT-3 doesn’t have human understanding, sure, but it has some form of understanding, absolutely. GPT-3 essays have been included in The Best American Essays anthology and it can write a serviceable undergraduate term paper (the term paper example is something even Gary Marcus has said, I guess he just finds this accomplishment unimpressive). It can even help with PhD theses.


Marcus’ predictions of faults and limitations regarding the GPT series (which have almost entirely been overcome with the later models) has been chronicled by Gwern and more recently Scott Alexander also detailed them (followed by an exchange with Marcus you can read here).
Importantly, GPT-3 and other “chatbots” are nowhere near the endpoint of this technology. The new PaLM from Google looks even more obviously a proto-attempt at AGI, yet another of these “foundation models.” PaLM cost 17 million dollars in compute power just to train (and that’s not including the research, the engineering, people’s salaries, testing, etc), clocking in with a neural network of 540 billion parameters.
PaLM’s abilities have been assessed across a set of 150 language modeling tasks, things like “Given a narrative, choose the most related proverb” or “Identify whether a given statement contains an anachronism” or “Answer questions about causal attribution,” and also things like the verbal reasoning section of the GRE, which means it does well on questions like this:
Just a decade ago this would have been unthinkable, but Marcus says that “fundamentally current AI is illiterate.” PaLM itself isn’t available for public use, and critics have leaned on that. Are these the good test results that Google wants to share with the world? Yes. Are they faking that it can do all sorts of tasks and approach human level at answering questions that were once beyond the domain of machines? No (here’s the paper).
For instance, here is how current AIs perform on a set of 24 language tasks (a smaller version of the full 150, which is called “BIG-bench”). AI progress is measured on such benchmarks, which are large datasets of questions.
As you can see, these AIs do surprisingly well compared to the average human performance on a lot of these tasks. And yes, they’re still behind the best humans. But what I’ve never seen pointed out anywhere is that researchers always give the humans (“evaluators”) all sorts of advantages to these tasks.
In order to make this baseline as strong as possible, we encouraged evaluators to use all available resources (including internet search) when performing tasks. . . if a rater completed a task multiple times, we consider their average performance on the task. . .
Given the breadth of content within BIG-bench, spanning multiple languages (natural, programming, and synthetic), vast swathes of domain-specific knowledge, and varying amounts of assumed mathematical and scientific background, aggregating all of this evaluator effort into a single number representative of “human performance” on any given task is a fraught enterprise. For example, if a task requires knowledge of programming, how do we weight scores of evaluators who do not know how to program?
. . . Our raters spent between 30 minutes and 2 hours per day performing these tasks over a period of weeks, with task subsampling so that a task could be completed in the given time window.
In other words, the benchmark tests are really take-home tests for humans, wherein they could spend hours on their questions, and use whatever internet resources they needed, and if the humans couldn’t do a task (like programming, or speaking another language) they were just left out of the data set. AFAIK PaLM and these other AIs don’t have internet access for querying; when models answer domain-specific questions (like say, about geography or programming), it’s “off the top of their head.” And they answer almost immediately what the humans take hours to do.
Might these AIs occasionally say dumb things? Sure. Might they take a couple tries to get it right? Sure. Are some things beyond them? Yes. But progress is accelerating. Google recently released Minerva, a math AI, which moved the highest performance on a well-known benchmark of high school math competition questions from a mere 7% to over 50% (not regular math homework, competitive questions, and these aren’t true/false). Again, this is undeniable progress, past the 95th confidence interval of the most optimistic predictions of AI forecasters. In terms of breadth and speed these new models are unrivaled, and their abilities often improve as a log-linear function of model size. There is no evidence that we are anywhere near plateauing in terms of the gains of scaling up these techniques by feeding them more data and making them bigger.
Finally, back in July, under the blistering pace of benchmarks being shattered, Marcus grudging admitted that Minerva is “measurable progress on a benchmark,” while emphasizing that getting good on such benchmarks (non-multiple choice math questions that most people can’t even do) is not actually that impressive. This is only slightly less extreme than what he said in April about both PaLM and DALL-E together, which is that “neither represent progress” on the deepest challenges of the field.
Yet PaLM and DALL-E literally represent progress on the deepest challenges. Consider how, back in 2012, Marcus wrote in The New Yorker that such deep learning techniques
lack ways of representing causal relationships (such as between diseases and their symptoms), and are likely to face challenges in acquiring abstract ideas like “sibling” or “identical to.” They have no obvious ways of performing logical inferences, and they are also still a long way from integrating abstract knowledge, such as information about what objects are, what they are for, and how they are typically used.
All of Marcus’ predictions for limitations turned out to be wrong. Deep learning can now do all of those things. PaLM can perform logical inferences, including those about causation, GPT-3 can integrate abstract knowledge, including how things are used and what they’re for, DALL-E 2 has a lot of information about what objects are and look like, and so on. Are they as good as the best human? No. Can they do it? Yes. If going from basically-zero to still-worse-than-the-best-humans-but-better-than-some-humans on language, reasoning, and math benchmarks does not represent progress on the deepest problems of the field, what would?
Therefore, where AI critics always end up is simply clinging to the goalpost of judging AI by whether it is equivalent to the human mind. E.g., about GPT-3 Marcus asks:
But being grammatically fluent is one thing; being a good model for the human mind is another. How can one tell?
Yet, GPT-3 is obviously not a model of the human mind. Consider how this inappropriate frame impacts how he interprets the output of “AI-artists” like DALL-E 2 and Imagen.
His point being that the models prompted with “a horse riding an astronaut” returns only images of the (more sensible) astronaut riding a horse. Except DALL-E 2 actually can make this image:

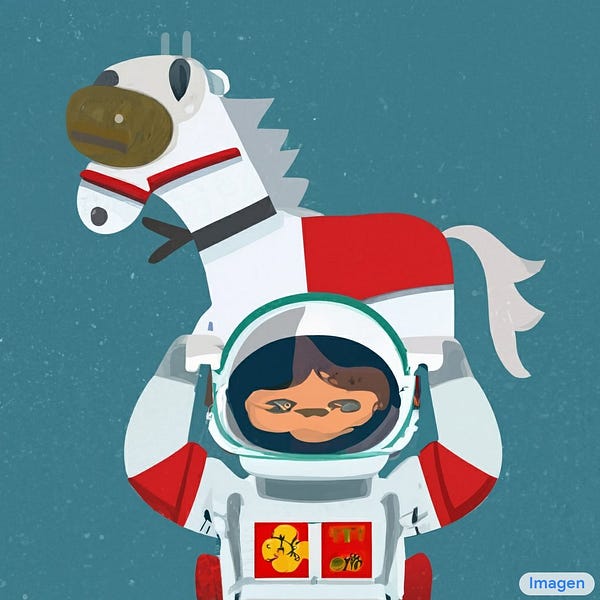
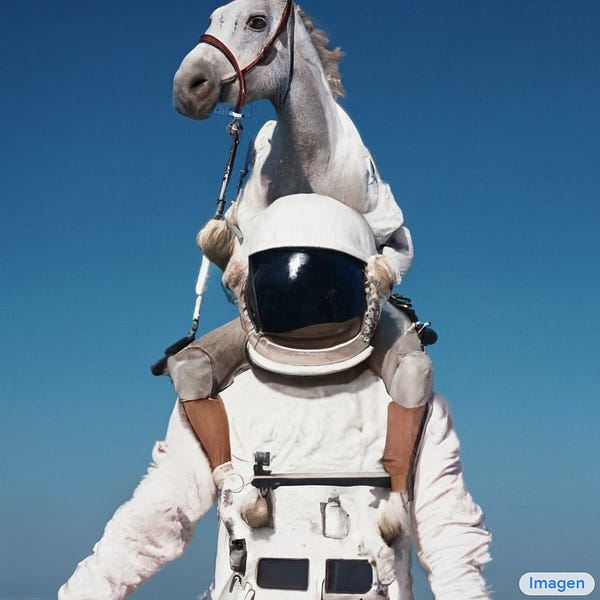

The above was generated by "A horse riding on shoulders of an astronaut" rather than Marcus’ attempted prompt “A horse riding an astronaut.” But to Marcus this failure of the model for the first prompt is an indication that the model is unintelligent or unimpressive.
Marcus’ criticisms operate via the assumption that a failed answer of a model is revealing of the model’s mental capacity in the same way that a botched answer would be about a human’s mental capacity. But that’s just not how these models work—their answers are not “psychologically revealing” about their mental capacity in the way a human’s answers are. It’s a category error. And that’s why fixating on the issue of reliability is also a category error. Humans think about what they’re going to write or paint, and it takes them a long time, and they really try to get it correct on the first go. Throw all that out the window for AIs. It’s easier for them to generate 10 images or text completions in a couple seconds, and yes, some, perhaps the majority, will be sub-human and stupid, but the others are often incredibly convincing, either in whole or in part. But then we say things like “only a 30% success rate” rather than “it gave at least one good human-level answer in under five seconds.” So the very background psychological assumptions we have, that people try to answer questions and, correspondingly, give singular answers they are confident in, and that therefore individual answers reflect people’s mental ability, are all totally off with AIs, who are better conceptualized as alien beings who are dedicated actors and willful liars, and who have no core of personality or singular world model at all. An example of this is how delicate prompting (what Asimov called “robopsychology”) can yield capabilities no one knew the model had.

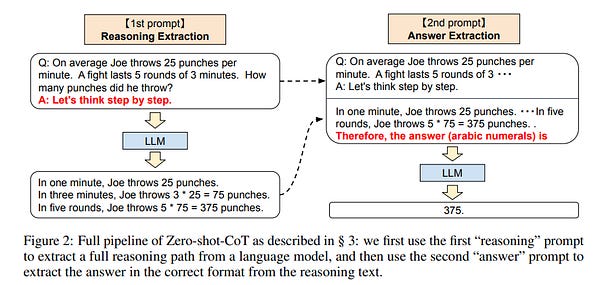
At this point, I’m sure Marcus would retreat from his bailey of implying deep learning is a parlor trick like an unintelligent horse that does fake arithmetic to the easier-to-defend motte where he’s just giving necessary criticism, noting these models aren’t perfect and still can’t do certain things, and suggesting that maybe there’s another path for human-like intelligence. And, sure, some of the stuff in the motte may be true! Maybe all of it is. But the stuff in the bailey belies the frightening rapidity of progress, and also rests entirely on the incorrect comparison to the reliability and honesty of the human mind. Which is obvious when you consider what Marcus said about such errors:
. . . anyone who is candid will recognize that these kinds of errors reveal that something is, for now, deeply amiss. If either of my children routinely made errors like these, I would, no exaggeration, drop everything else I am doing, and bring them to the neurologist, immediately.
Marcus, despite my singling of him out as representative of AI critics, is not alone. There are plenty of other AI critics, who often take the same tack. Consider Douglas Hofstadter, who wrote a piece in the Economist saying that these AIs are “cluelessly clueless.” Hofstadter makes exactly the same mistake as Marcus by giving single prompts, finding some silly answers, and assuming that this is deeply revealing of the model’s extremely alien psychology. Except slightly different prompts to Hofstadter’s supposedly revealing questions return human-level sensible answers (other critics use the same sort of conflations).
The ultimate longterm problem for the AI critic approach is that the standard of the human mind is an impossible goalpost. Neuroscience lacks the sort of big overarching theories it would need for us to clearly understand how the mind works. If someone says they do understand how the mind works, they are selling you something (like, say, a book, or a company). So we can’t a priori design the principles of the human mind into an AI. And we will have AGI, at least to some degree judging from current progress, long before we understand how cognition works in real brains. So then what’s actually interesting about these models, and AI in general, is how smart it is in the space of possible intelligences without being like human minds. In a cartoon picture this looks something like:
The problem is that, in our world, we do not know what this space really looks like, how much overlap there is, etc. We do not know its dimensionality, we do not know how far it goes. So we plod around in waters of unknown depth.
A strange thought. In a perverse way, the amorphous, unreliable, and inhuman nature of AI minds has ended up being the perfect shield for their development. It’s a schizophrenic aegis that decouples criticism from their actual capabilities. AI’s critics, so focused on judging these things as if they’re humans, delay everyone from taking these new technologies as seriously as we all need to. In a weird twist, AI’s own critics serve the AIs in this. For, hidden amid the mud stirred up by critics’ protestations, corporations continue to pump out nascent alien minds that are ever more inhuman and ever more artificially intelligent.
Also: ask one hundred humans to draw a horse riding an astronaut & I bet about fifty of them would draw an astronaut riding a horse.
Interesting post. I have to say I had been very sympathetic to Marcus but this post makes me less so.
Something that leaves me somewhat sympathetic to Marcus’ rhetoric calling these “parlor tricks” and such is the fact that these models, while superficially impressive, have yet to yield much value in real world applications. I realize for PALM and Dalle-2 it’s still super early, but we’ve had GPT-3 for years and as far as I know there have been no major applications of it beyond “hey look how novel it is that an AI wrote part of this article”. Whenever I talk to people who program/use AI in commercial applications, they are much more cynical about its capacities.
Given that, it really does seem like something is missing, and maybe we’re prone to overestimating these models. While many of the specific critiques made by Marcus were wrong, I think he’s getting at that more general intuition.
As a side point, I’m also more skeptical than Erik is on how much progress we’ll get for further scaling, given that we may be running out of the kind of data these models use to train: https://www.lesswrong.com/posts/6Fpvch8RR29qLEWNH/chinchilla-s-wild-implications