The #1 AI can't teach basic stuff like reading
Claude Sonnet 3.5 fails miserably at preschool lesson planning
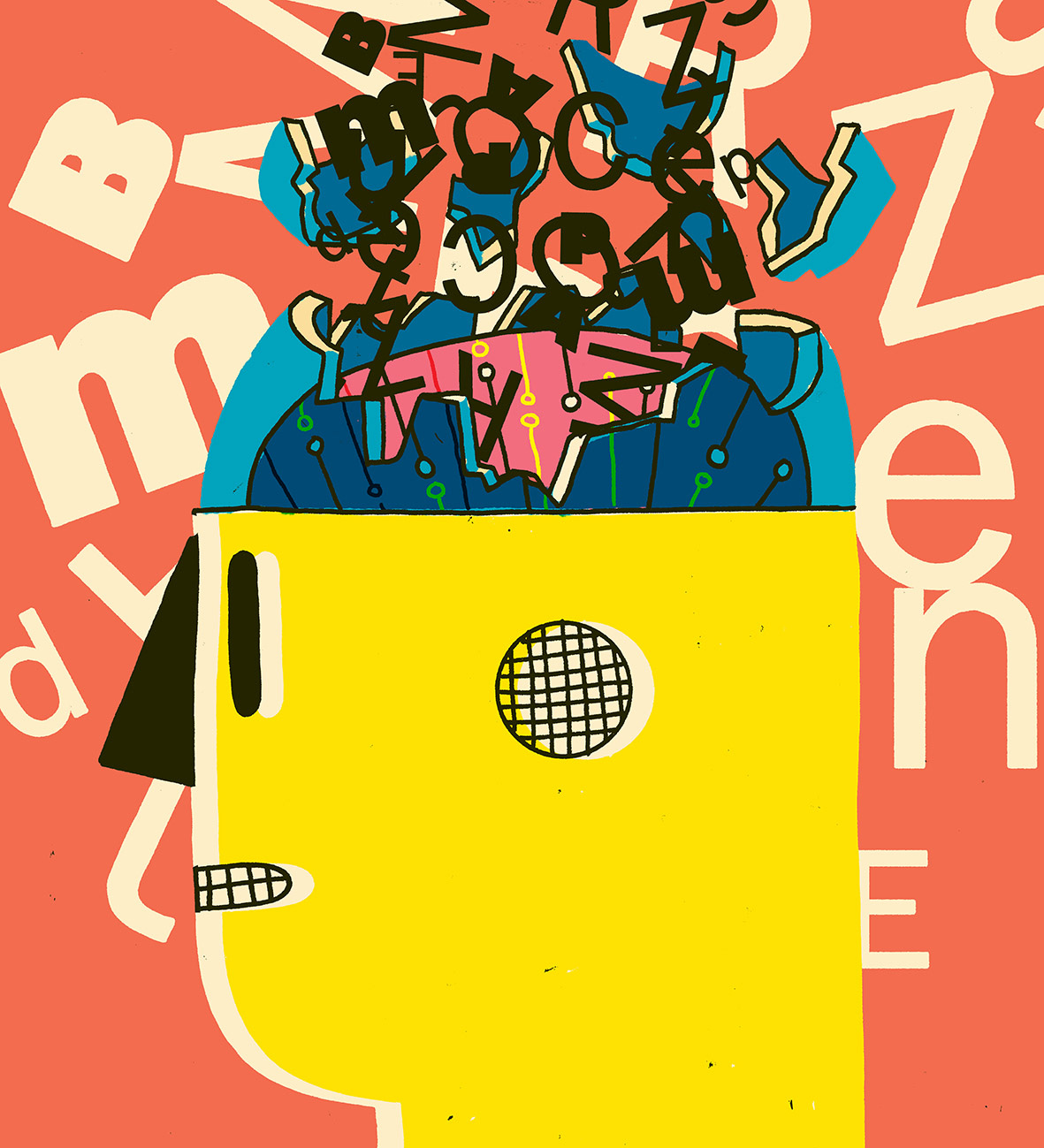
In theory, I’m someone who could use AI assistance. Not for actual writing, of course, since that would remove the meaning of my work. Such a swap would be like passing off everything I do to some encyclopedic but banal ghost writer I can only email with.
No, I mean that if the newly-pivoted-to goal of Silicon Valley is to add hirable surplus intelligence to the world, there’s certainly a set of things I’d be comfortable ethically hiring intelligence for, from asking an AI “What are the most interesting recent papers on causal modeling in complex systems?” to “Can you check this essay for any odd grammatical mistakes I didn’t notice by my third read-through?”
And yet I find myself unable to make use of the widely-hyped surplus intelligence that’s available. There’s a strange disconnect. Nvidia (the major manufacturer of the chips used to train AIs) was just the most valuable company in the world. People claim that leading models, like Claude Opus, can write legal opinions as good as Supreme Court Justices. And now there is an even better model than that, Claude Sonnet 3.5, out this very June. I mean, look at the rate of progress on benchmarks of intelligence!
And yet. Whenever I try to use AI “in the wild” to actually assist me at even trivial tasks, it fails. There’s an uncanny gap wherein during testing, even my own, it looks impressive, and other people sure seem to find it impressive (except Gary Marcus), but whenever I deploy it for a real purpose it falls short.
Consider one much hyped use-case: supplemental AI tutors in education. Yesterday, The New York Times reported on the boondoggle of an AI chatbot “Ed,” commissioned for 6 million dollars by the LA school district for its classrooms (the company, in turn, collapsed). AI tutoring is the goal of a number of startups, like Synthesis, an experimental school originally started with backing from Elon Musk. Synthesis has pivoted to an education app integrated with AI (recently raising 12 million in funding).
Another example is Mentava, an app in spirit close to my heart, since it’s designed to help young kids learn to read (especially the very young). Mentava got a lot of attention earlier this year when some troubled soul discovered their pitch deck and was very mad about… kids learning to read? This led to a wave of people purchasing their beta product, a $500 app that is supposed to teach a child to read (unclear to me how this can work without a parent there to correct inevitable mistakes, but I digress).
The explicit dream of such “edtech” is “The Young Lady’s Illustrated Primer” from Neal Stephenson’s novel Diamond Age—essentially an AI tutor that takes a destitute young girl and molds her into a sovereign. Yet, are even the very best AIs anywhere near taking on the role of a teacher? It’s an easy question to answer, since there’s a definite initial hurdle they need to pass. The first step of any official education is learning to read. If an AI can’t reliably help teach reading (not even do it by itself, just help) it’s unclear what Large Language Models are actually good for in education. Presumably, steps after reading get harder, not easier. And I’ve discovered, even just for writing basic lesson plans, that…
The leading AI fails spectacularly at replacing the role of a first-grade or even preschool teacher.
As I’ve written about in an ongoing series, I’ve been teaching my three-year-old son to read. My method is to start with the most common letter sounds. A like the start of "apple.” B like “bite.” C like “cat,” not “cent.” Once the child knows the 26 most common letter sounds, then you can start complexifying. You can come up with words that only use those most common sounds (e.g., “bob” but not “boat"), then come up with entire sentences (e.g., “bob sat up”), and finally start adding on extra rules (e.g., that ch together is pronounced differently—like “chin”—than c and h are separately).
Now, just like with my writing, I wouldn’t pass the teaching of my son off to an AI. But I could, in theory, make use of AI to prepare parts of lessons. That would be a great use of an intelligence surplus. So that’s precisely what I recently tried to do with Anthropic’s new Claude 3.5 Sonnet, their most powerful model. One that is, according to test scores, better than anything else out there.
Can you guess what happened? Mr. Sonnet failed terribly when assisting for lesson planning for a literal three-year-old.
Specifically, one of the lessons I do (there are others of different kinds, like Beginning Readers books) is creating new simple stories that I can put onto an iPad and have my son read (on an iPad you can change the font size, since he finds small text harder to focus on). To do that, I need to think of three sentences that only use the most common letter sounds, like: “Bob ran fast. Bob slips in mud. Bob got up.” Coming up with these is no great mental feat, and it’s tedious. It would be helpful to get a couple dozen example sentences at a request instead of thinking of them all myself. A real actual AI use-case. So I asked Sonnet precisely this:
I want to emphasize that Sonnet appears to perfectly understand what I asked for. It first gives a correct summary of the request, but then the results are dismal (feel free to skip reading 100%, I’ll explain below).
Incorrect words that don’t match the request include: “tried,” “pail,” “eat,” “liked,” “yellow,” “shop,” “night” (come on!), “lollipop,” “with,” “splash,” and more. Additionally, “the dog did not bit the cat” is not even grammatically correct to begin with (should be “bite,” which would also be wrong since the silent e is not the most common sound).
There’s even more basic stuff wrong, like “the” and “was” or even stand-alone letters like “a.” Now, my kid could read those anyway, since he knows some common sight words (words kids know by sight but not phonetically). He also knows the rule that letters alone are just their names, and also when consonants repeat you usually just say it once (e.g., “nn” is the same as one “n”), and some other minor rules as well. You might charitably think that Sonnet assumed a background knowledge of common sight words (which I do sometimes use) as part of the request. Does that explain mistakes like “lollipop” or “night?” Absolutely not.
Perhaps Sonnet, despite summarizing the directions perfectly, didn’t actually understand? I tried reminding it about what constitutes the most common letter sounds.
Its accuracy here is unsurprising, since there are a huge number of texts in its training set that say some version of this. But when asked to go back and apply this knowledge to how it messed up, Sonnet gets even more confused. First, it says it made “a mistake” in the original request (just one?). But then, when giving replacements, it makes not only a bunch of new mistakes, but the exact same mistake it identified!
Above, Sonnet is correct that using “splash” was wrong. Except the replacement sentence it gives has “saw.” Which is also wrong. But okay, let’s just push through. Maybe it will get more correct after this reminder?
Nope. Most of these stories have incorrect words yet again: “with,” “cold,” “them,” “his,” “hike,” “liked,” “napped,” “drove",” “of,” “noon,” “food,” “to,” “sea,” “tree,” and so on. Like, excuse me Mr. Sonnet, but you just said “splash” is not appropriate and next you go and use “fish”? It’s the same thing! That you just said was wrong! It’s almost as if…
Sonnet is kind of an idiot.
There’s been a long trope that critics of AI have made use of, which is that 97% performance is radically different than 100% performance (just think of self-driving cars). This applies to way more tasks than at first seems obvious. While it’s not launching a rocket, you can’t be teaching a child to read and not getting the sounds right. That would be devastatingly confusing. Kids pick up on things so quick that if you teach a mistake you’ll have a hell of a time undoing it.
Now, I’ve tried this with different prompts. I’ve asked it to list out all the letter sounds with examples of each sound (which it can do) and then create sentences. Nothing worked for me. Of course, I can’t rule out that there’s some prompt out there somewhere that works, and that’s not my claim. In fact, let’s say that someone created some working prompt with a ton of background context and carefully structured examples. Does it obviate my criticisms here? I think not, for a few reasons. First, even assuming there turns out to be some hidden hyper-specific recipe out there in prompt space that can churn such sentences out, small deviations, like simple follow-up questions, would still likely break it down (this is a criticism I have for most “solutions” to tasks AIs struggle with, which is that they are unstable solutions). Second, clearly the model understood the request in the sense of being able to repeat it back. It also understood what it did wrong. A human in that epistemic state would not make the same mistake twice, using “fish” immediately after “splash” was acknowledged as incorrect. Third, in a teaching environment the prompts you’re receiving are from kids! They have no idea what they’re doing, or asking, or saying (in the best way). To be blunt about it: the spiraling loop of confusion between a child trying to learn to read and even a hundred-billion-dollar AI right now would be a true sight to behold.
If the leading AI can’t help me with lesson planning for a literal three-year-old toddler… perhaps there’s truth AI is going through a hype bubble. In a last grasping at straws, one might wonder if the failures for phonics is specific to the fact that sounding out words goes beyond just the relations in language alone. Yet if that objection is true, think of the generalized case: everything is like that. Sonnet is limited in the same way it is for pronunciation as about essentially all subjects for which it has no real experience. It has no mouth, yet it must help.
Or to put it in a three-sentence story using only the most common letter sounds:
Sonnet must help. Sonnet did not help. Bad Sonnet, bad.
The state we are in right now is what Ethan Mollick has called the "jagged frontier", where the large-language models are substantially better than the average human at many tasks, and substantially worse than the average human at many others, and you cannot tell without experimentation.
I'm glad you are actually using an AI system to try (if unsuccessfully) a use case. And I'm especially glad to see that you tried multiple prompts. Too much AI scorn and hype comes from single attempts, without either experimentation to improve performance, or repeated trials to see whether the result can be achieved consistently. It's very much an in progress technology; I wouldn't want to make a substantial bet on what it can or cannot do 18 months from now.
The problem with Gen AI is that people keep getting lulled into thinking they are working with a thinking, knowledgeable system. They keep forgetting how Gen AI works. It's only a prediction machine. It's predicting what based on its algorithms the most likely next word. When it gets it right or close to right we are amazed. When it gets it wrong we call it an "hallucination". In reality it's always hallucinating. We just like the results a lot of times. We have to keep it mind that even though it uses words to try and reassure us it does not truly understand. It's just predicting and repeating based on what has been previously written. So what good prompters do is figure out how to make it predict better, not make it understand.