AI progress has plateaued below GPT-5 level
Or, “Search isn’t the same thing as intelligence”
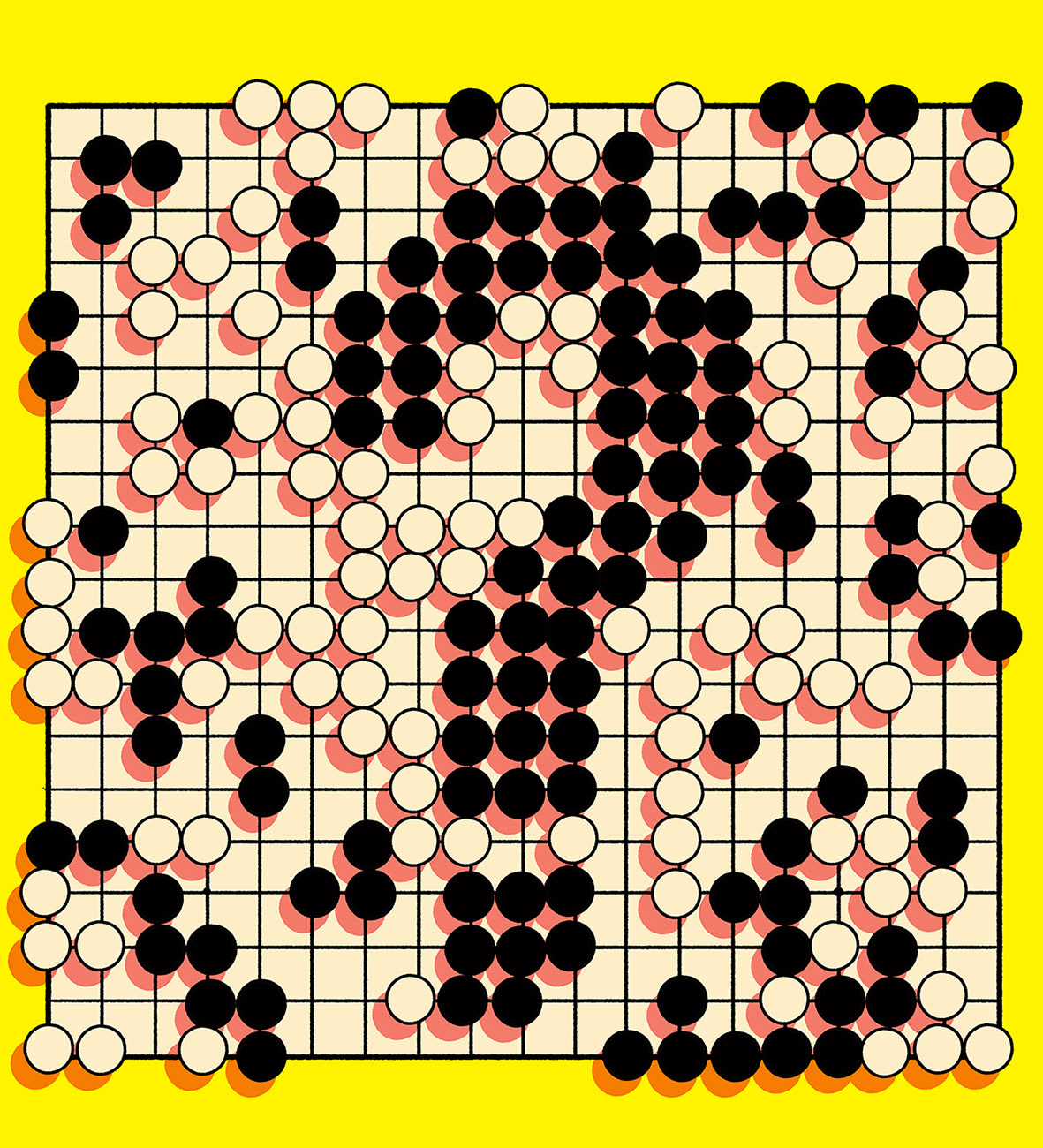
According to inside reports, Orion (codename for the attempted GPT-5 release from OpenAI) is not significantly smarter than the existing GPT-4. Which likely means AI progress on baseline intelligence is plateauing. Here’s TechCrunch summarizing:
Employees who tested the new model, code-named Orion, reportedly found that even though its performance exceeds OpenAI’s existing models, there was less improvement than they’d seen in the jump from GPT-3 to GPT-4.
If this were just a few hedged anonymous reports about “less improvement,” I honestly wouldn’t give it too much credence. But traditional funders and boosters like Marc Andreessen are also saying the models are reaching a “ceiling,” and now one of the great proponents of the scaling hypothesis (the idea that AI capabilities scale with how big they are and the amount of data they’re fed) is agreeing. Ilya Sutskever was always the quiet scientific brains behind OpenAI, not Sam Altman, so what he recently told Reuters should be given significant weight:
Ilya Sutskever, co-founder of AI labs Safe Superintelligence (SSI) and OpenAI, told Reuters recently that results from scaling up pre-training—the phase of training an AI model that uses a vast amount of unlabeled data to understand language patterns and structures—have plateaued.
I know that there is a huge amount of confusion, fear, and hope that creates a fog of war around this technology. It's a fog I've struggled to pierce myself. But I do think signs are increasingly pointing to the saturation of AI intelligence at below domain-expert human level. It’s false to say this is a failure, as some critics want to: if AI paused tomorrow, people would be figuring out applications for decades.
But a stalling makes sense because, honestly, we haven’t yet gotten a model that feels significantly smarter than the original GPT-4 (released early 2023). Sure, there have been some advancement on the benchmarks, but there’s been no massive leap in real-world performance other than (a) an increase intelligence to at best an occasional “GPT-4.5ish” level, but not a GPT-5 one, (b) a useful decrease in hallucinations, and (c) a pleasing task-relevancy of responses (but we wait longer for them). How much this matters to users depends greatly on the task. E.g., metrics like the ranked persuasiveness of AI-generated political messages show sharply diminishing returns with model scale, such that leading frontier models are barely more persuasive than order-of-magnitude smaller ones.
Here’s the successive increases in official performance on the flagship Massive Multitask Language Understanding benchmark (the MMLU is a bunch of hard tasks ranging from math to law). At first it looks impressive, but if you look close no model has made significant progress since early 2023.
And we now know that models are often over-fitted to benchmarks. As Dwarkesh Patel wrote while debating (with himself) if scaling up models will continue to work:
But have you actually tried looking at a random sample of MMLU and BigBench questions? They are almost all just Google Search first hit results. They are good tests of memorization, not of intelligence.
One issue with discerning the truth here is that researchers who release the models aren’t unbiased scientists, so they don’t test on every single benchmark ever. They are more likely to choose benchmarks that show improvement in the announcement. But I’ve noticed online that private benchmarks often diverge from public ones. E.g., independent researchers and users were less impressed by this summer’s GPT-4o:
I think it’s likely that over the past year private benchmarks show more variable performance (e.g., not always going up across the models) compared to the public benchmarks everyone uses (this often appears to be the case). In other words, improvements look ever more like “teaching to the test” than anything about real fundamental capabilities.
In which case, it’s pretty meaningful news that models like Orion/GPT-5 are not improving universally across the tests like they used to. As a recent article in The Information revealed:
Some researchers at the company [OpenAI] believe Orion isn't reliably better than its predecessor in handling certain tasks, according to employees. Orion performs better at language tasks, but may not outperform previous models at tasks such as coding, according to an OpenAI employee.
Another sign of stalling is that, despite all the hype, companies like OpenAI are now regularly not releasing internal models to the public, almost certainly because they don’t work as well as the hype claims. For instance, the simple explanation for why OpenAI’s video-generating AI Sora isn’t publicly available—after nearly a year!—is because it sucks. If OpenAI did release Sora it would be met with mostly laughter and mocking. As I argued in “Recent AI failures are cracks in the magic,” the information density of video makes it very obvious when the AI gets things wrong (we humans have an entire lobe of our brain dedicated to picking out the slightest visual errors).
In addition to keeping some models locked behind closed doors, recent deployed models, like GPT-4 Turbo or GPT-4o, are likely already-failed attempts at GPT-5, and have consistently been stuck at the same roughly “GPT-4.5ish” level (which is what Turbo and 4o and now 4o1 basically are). Many have speculated that the expectations are so high that OpenAI keeps renaming failed GPT-5 attempts as “GPT-4-whatever” to not expend the built-up hype (in fact, they may never release a GPT-5, just keeping Orion as “Orion” when it launches next year).
Why is this stalling out happening?
The first hypothesis was given during the initial wave of criticism against deep learning: that these systems lack symbolic reasoning and therefore couldn’t ever “truly” reason. These objections have turned out to have far less teeth than predicted—e.g., Gary Marcus used to lean heavily on the idea that AIs can’t do causal reasoning because they’re just “curve fitting,” but they obviously can reason about causation now. At the same time, the models never quite overcame their nature as autocompleters, still getting fooled with simple questions like “How many Rs are in strawberry?” or not being able to generate simple phonics lessons.
The second hypothesis behind the stall is one people discuss all the time: deep learning is limited by the amount of high-quality data (of which human civilization has been thoroughly mined of by the big companies). This creates an “S-curve” that, at best, approximates human intelligence on tasks with lots of data (I’ve pointed out this entails a “supply paradox” wherein AI is good at things that aren’t that economically valuable at a per-unit level, like writing text). That is, they are essentially just a function of the data that made them (explaining why AIs converge to be so similar).
Of course, there’s still the question of whether or not synthetic data can get around this. But there are well-known downsides, like model collapse, and this seems the sort of obvious solution that I suspect the companies have been trying to not much success.
Why AI is stalling out is probably some combination of the two standard reasons. There was always some sketchiness to the degree of which deep learning was mimicking real human reasoning (but critics called “the wall” early by several orders of magnitude). And this sketchiness could be papered over, but only via huge amounts of data, which these companies have run out of.
Perhaps we should not dwell too closely on the question of why it is that the sum of human civilization’s data fed into deep learning techniques plateaus at something smart—but not that smart—lest we see ourselves too clearly in the mirror.
What to make then, of the claim that researchers have unlocked a new axis of scaling by giving the models time to “think”? For this is what the entire industry will now rely on, both in practice but also to keep the sky-high hype alive.
I think this new axis of scaling will matter far less than people expect and will not keep the train of improvements chugging along anywhere near the previous rate.
First, this new type of scaling appears limited to certain domains: it makes the models no better on English language tasks or provides only minimal improvements for questions about biology, for instance. Second, there are good reasons to believe that this sort of scaling will be much more expensive: linear improvements for extreme sums of money. Here’s OpenAI’s chart on this new scaling law (shown only for a math benchmark, notably), released alongside their most-recent 4o1 model from September:
As tech journalist Garrison Lovely wrote about it on Substack:
If you have a way of outcompeting human experts on STEM tasks, but it costs $1B to run on a days worth of tasks, you can't get to a capabilities explosion, which is the main thing that makes the idea of artificial general intelligence (AGI) so compelling to many people…. the y-axis is not on a log scale, while the x-axis is, meaning that cost increases exponentially for linear returns to performance (i.e. you get diminishing marginal returns to ‘thinking’ longer on a task). This reminds me of quantum computers or fusion reactors—we can build them, but the economics are far from working.
But I think people focusing on price or the domain-specificity of improvements are missing the even bigger picture about this new supposed scaling law. For what I’m noticing is that the field of AI research appears to be reverting to what the mostly-stuck AI of the 70s, 80s, and 90s relied on: search.
I don’t mean searching the web, rather, I mean examples like when this summer Google DeepMind released their state-of-the-art math AI able to handle mathematical Olympiad problems. It's a hybrid, very different than a leading generalist LLM. How does it work? It just considers a huge number of possibilities.
When presented with a problem, AlphaProof generates solution candidates and then proves or disproves them by searching over possible proof steps…. The training loop was also applied during the contest, reinforcing proofs of self-generated variations of the contest problems until a full solution could be found.
This sort of move makes sense, because search was the main advantage for AIs against humans for a very long time (e.g., in Chess, whereas Go cannot be brute-forced in a similar manner). But AI relying too much on search has been criticized before. In the infamous Lighthill Report in 1973, James Lighthill pointed out that combinatorial explosions awaited any AI techniques that relied on search—predicting well the limits of the field for decades until the advent of deep learning (which actually made progress on real intelligence).
I think the return to search in AI is a bearish sign, at least for achieving AGI and superintelligence. This is for a very simple reason: search over a vast number of possibilities is not at all like human thought! Nor is it qualitatively the same as having one singularly smart big artificial neural network (like a big super brain). Rather, it is merely a coupling of the traditional superiority of computers—search over huge numbers of possibilities—with some sort of roughly-GPT-4.5ish-level intelligence that mainly just proposes and evaluates.
In fact, the entire new axis of scaling touted by the companies to replace the old failing scaling laws is arguably just search given a makeover (Noam Brown, a foundational contributor to GPT-4o1, describes his research mostly in terms of advances in search). As Reuters reported a few days ago:
To overcome these challenges, researchers are exploring “test-time compute,” a technique that enhances existing AI models during the so-called “inference” phase, or when the model is being used. For example, instead of immediately choosing a single answer, a model could generate and evaluate multiple possibilities in real-time, ultimately choosing the best path forward.
People (both in the field, but also the writers for Reuter) are keen to describe this sort of thing in mentalese. Yet actually, both the leading avenues—“test time training” and “test-time compute”—look an awful lot to me like just fancy search!
In “test-time training” the model rapidly adjusts its own parameters (like fine-tuning) in order to find the parameters best suited for that particular test. In other words, it searches over parameter space. In “test-time compute” there are hidden iterative refinement stages to the official output where multiple draft outputs are evaluated or averaged or voted on. Either way, these methods require more and more time and more and more resources in order to explore possibilities, and no matter how much search you do you’re still fundamentally bounded by the place you started, which is converging across models to some sort of roughly GPT-4.5ish level intelligence.
Some version of search is what researchers are leaning on everywhere now; e.g., recent gains on the ARC test, a test of general intelligence considered difficult for LLMs that had a jump in performance just a few days ago, were caused by using a similar kind of “test-time training.”
Continued improvements are going to happen, but if the post-GPT-4 gains in AI came mainly from adding first better prompts (chain-of-thought prompting) and now more recently the addition of search to the process (either over many potential outputs or over the model’s parameters itself) this is different than actually constructing baseline-smarter artificial neural networks. It indicates to me a return to the 70s, 80s, and 90s in AI.
A mini thought experiment. Who do you think can make breakthroughs in physics? One Einstein or 1,000 physics undergraduates? Barring that none of the undergraduates just happens to be an Einstein-in-waiting, the answer is one Einstein. We already know that very large numbers of people are rarely as good as a dedicated small team or even a lone individual in terms of creativity, knowledge, and impact. These new scaling techniques are the creation of an algorithmic pipeline that gives better answers via “cloning” or “varying” an already-saturated-near-max intelligence.
Does this new reliance on search-based techniques obviate all concerns about AI safety and existential risk from these technologies? No, it doesn't. Pandora's box has been forever unlocked. Forevermore, we will live with the existential risk of AI. However, the news that baseline general intelligence is plateauing, and that AI researchers are now relying on old standbys like massive search spaces to progress, is an early indication that—at least barring further fundamental breakthroughs—timelines have just been pushed back in our lifetimes.
For the sake of human culture not being filled with unconscious AI slop, for the sake of our children being able to find meaning in their own expertise and abilities and intelligence, and for the sake of risk-blind primates who thought it a good idea to try to make entities more intelligent than themselves—I personally hope I'm right about a return to search being bearish. I can’t offer much more than that.
I hope “big tech” keeps chasing the “AI” mirage long enough to do a big nuclear power build-out, then, when “AI” collapses, make all that cheap energy available to consumers.
"For the sake of human culture not being filled with unconscious AI slop, for the sake of our children being able to find meaning in their own expertise and abilities and intelligence, and for the sake of risk-blind primates who thought it a good idea to try to make entities more intelligent than themselves—I personally hope I'm right about a return to search being bearish. I can’t offer much more than that."
Btw, I want to add how much I love you for your pro-humanity. You see so few people these days willing to wear that badge with the pride and the purity you do.
Thank you, Erik.